From capacity constraints and workforce shortages to escalating costs and changing customer expectations, the rail industry stands at a crossroads. As in other industries, artificial intelligence (AI) has the potential to help rail meet systemic challenges and transform the industry.
To understand how the rail industry sees the challenges and success factors involved in implementing AI, we recently conducted a survey among 50 industry participants across rail operators, infrastructure managers, original equipment manufacturers, and Tier 1 suppliers in more than 10 countries (mostly European). Survey participants ranged from C-suite level and founders to heads of technology, digital transformation, strategy, operations, sales, and supply chain.
Systemic rail industry challenges
The rail industry is grappling with a series of challenges that require strategic foresight and innovation. Although specific challenges will vary by country, there are some common denominators. For example, in many geographies, network and fleet capacity constraints are casting a shadow over rail’s ability to meet growing demand. Ensuring a sound decision-making process on infrastructure expansion, upgrades, and fleet modernization is a strategic imperative.
Skilled workforce scarcity and labor shortages are another key issue for many rail operators and suppliers, necessitating a nuanced approach to strategic workforce planning, labor automation, staff planning, as well as utilization and implementation of innovative employee initiatives and training.
Furthermore, the escalating costs associated with maintaining assets and infrastructure are a pressing concern, one that requires the industry to seek proactive solutions. Maintenance processes require increasingly complex planning and dispatching efforts. The trend towards preventive and predictive maintenance is a key opportunity but requires more stable data and data models. Third-party players are entering the market with increasingly complex financial models.
In addition, service level expectations for freight shippers and passengers are increasing, at the same time that the industry seeks to maintain cost efficiency. Enhancing both stability and flexibility will be essential, requiring the industry to harness real-time data, expedite information processing, and deploy agile rescheduling capabilities. In particular, rail needs to double down on increasing forecast accuracy around estimated time of arrival (ETA) for freight shipments. This would help meet passenger expectations regarding continuous improvement in the personal travel experience, and freight shipper demands for greater visibility into the location and transit time for cargo.
AI solutions for rail transformation
With its transformative capabilities, AI is emerging as a potential game changer for the rail industry. Along the entire value chain, from operations planning to customer services, AI offers solutions that promise to improve service levels and efficiency. To highlight a few examples:
Operations planning and dispatching
AI-driven planning strategies can optimize efficiency and responsiveness, ensuring optimal resource allocation and reducing operational bottlenecks. Real-time dispatching optimization could empower the industry to adapt swiftly to dynamic conditions.
Asset management and maintenance
Predictive and preventive maintenance, supported by AI, could revolutionize asset management. The ability to predict maintenance needs, dispatch trains for maintenance, and incorporate AI support during maintenance and repair would increase asset utilization and reduce downtime.
Autonomous driving technologies
AI could take center stage in autonomous driving technologies, offering solutions to overcome current technical limitations. The integration of AI could enable a seamless transition to autonomous systems while maintaining safety standards (if permitted by a country’s regulatory regime).
Customer services along the journey
AI could enhance the overall customer experience by facilitating travel instructions, individual on-board services, live updates, and improved customer communication. This not only could help meet evolving customer expectations but also position the rail industry as a more customer-centric service provider.
Road ahead for AI in the rail industry — challenges and success factors
Rail industry participants in our survey were asked to rank the challenges and success factors covering three main dimensions: technical, human, and other, as shown in Exhibit 1.
According to survey respondents, the primary challenge in today's landscape is securing high-quality data. Respondents ranked this factor as surprisingly more significant than other challenges. This may seem counterintuitive, considering the difficulties that rail and other transportation sectors have faced with digital migration in the past – often struggling to implement new systems within their organizations and gaining buy-in. However, it could indicate that the AI transformation is still in its early stages and focused on establishing the necessary prerequisites, such as ensuring the availability of good quality data and dealing with a multitude of legacy systems (Exhibit 2).
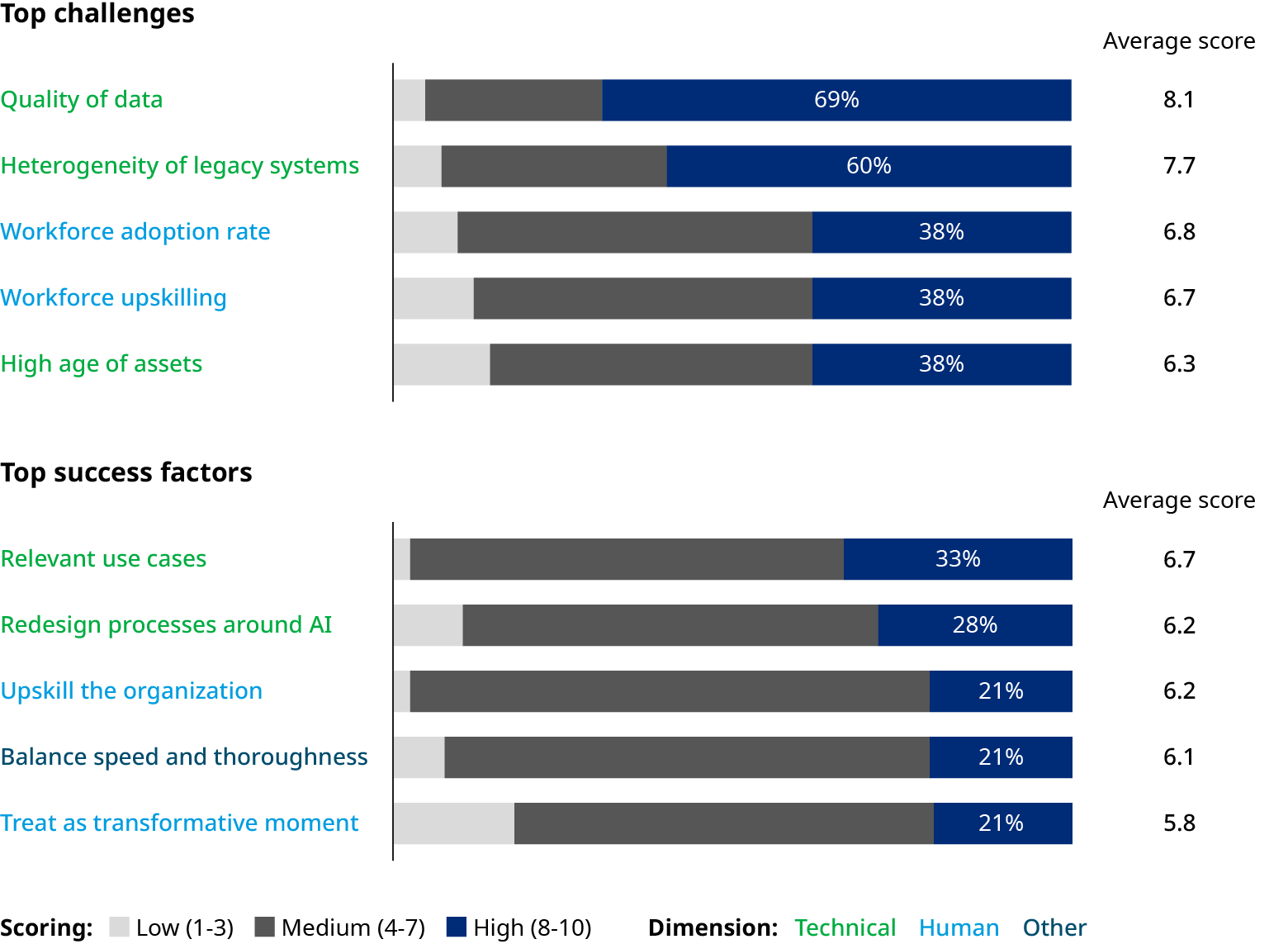
Consequently, respondents also prioritize technical success factors, such as finding the right use cases, over change management and human-related factors. Still, the overall picture for success factors appears more balanced across the three dimensions as compared to that for the key challenges identified.
Charting a course for an AI future in rail
Based on our experience with recent digital and technology migrations in the rail industry, we have found that the most significant challenge and crucial success factor is ensuring a high adoption rate. It is not sufficient to initiate the upskilling and change management process after developing a solution; rather, these need to commence as soon as potential use cases have been defined. People need to be involved in the definition and development of solutions through an integrated process that combines technology and change management. Taking shortcuts typically leads to low adoption rates, jeopardizing the entire AI transformation.
Additionally, the rail industry should consider greater collaboration within the industry, as well as leveraging technology and lessons learned from other industries that are more advanced in AI transformation. This approach would help mitigate some of the risk associated with in-house development and expand benefits to a broader community. And in turn, this could drive faster implementation of AI-enabled processes and enable management resources to focus on bringing the workforce up to speed.